Table Topic Integration with Tencent Cloud Iceberg
AutoMQ Table Topic supports seamless integration with Iceberg to enable streaming data lake analysis and querying, eliminating the need for ETL configuration and maintenance. This article outlines how to configure the integration between Table Topic and Iceberg in the Tencent Cloud environment.
Prerequisites
Using the AutoMQ Table Topic feature in Tencent Cloud requires meeting the following conditions:
-
Version Constraints: The AutoMQ instance version must be >= 1.4.1.
-
Instance Constraints: The Table Topic feature must be enabled when creating the AutoMQ instance. It cannot be enabled after the instance has been created.
-
Resource Requirements: Using the Table Topic on Tencent Cloud requires an existing Hive Catalog service. Users can purchase Tencent Cloud's managed EMR HMS service.
Steps
Step 1: Configure the Hive Catalog Service
You can configure the Hive Catalog on Tencent Cloud by setting up the Hive Metastore service yourself or by purchasing Tencent Cloud's managed EMR HMS service. This documentation uses Tencent Cloud's managed EMR HMS service as an example to illustrate the configuration method.
- Go to Tencent Cloud EMR Product, purchase a cluster, and enable Kerberos authentication.
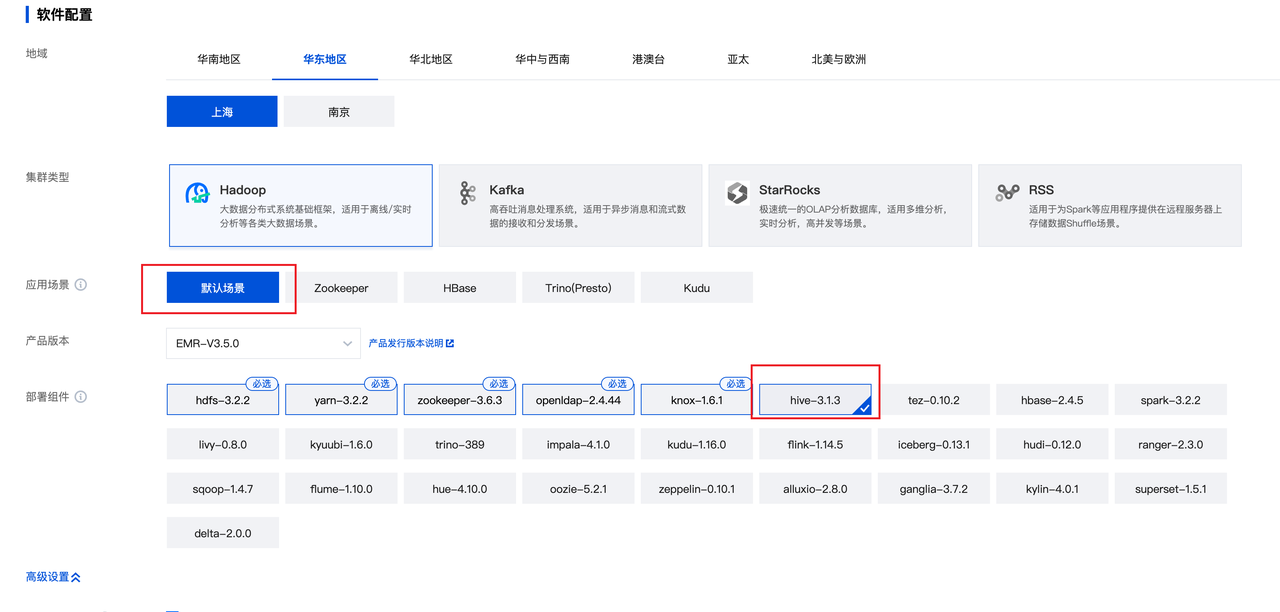
- Configure the HMS environment variables script hive-env.sh to add the JAR for accessing object storage.
# Add the JAR Dependency to Enable HMS to Access Object Storage.
# Hive-env.sh
HIVE_AUX_JARS_PATH=/opt/apps/HADOOP-COMMON/hadoop-current/share/hadoop/tools/lib/aws-java-sdk-bundle-1.11.375.jar:/opt/apps/HADOOP-COMMON/hadoop-current/share/hadoop/tools/lib/hadoop-aws-3.2.1.jar
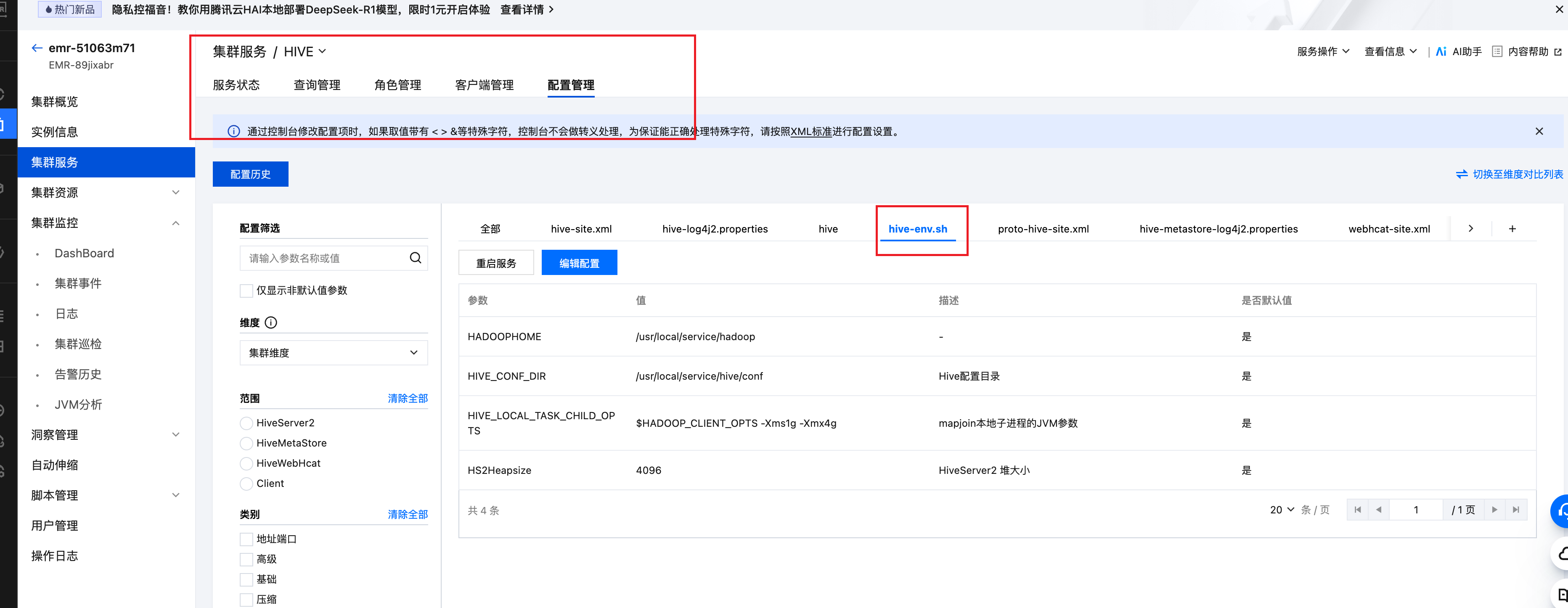
- Modify hive-site.xml to add the following object storage access configurations.
# Add Object Storage Access Configuration
# Hive-site.xml
fs.s3a.endpoint=https://cos.ap-shanghai.myqcloud.com
fs.s3a.access.key=$access_key
fs.s3a.secret.key=$secret_key
fs.s3a.impl=org.apache.hadoop.fs.s3a.S3AFileSystem
- After configuring the parameters, restart the Hive Metastore service to apply the changes.
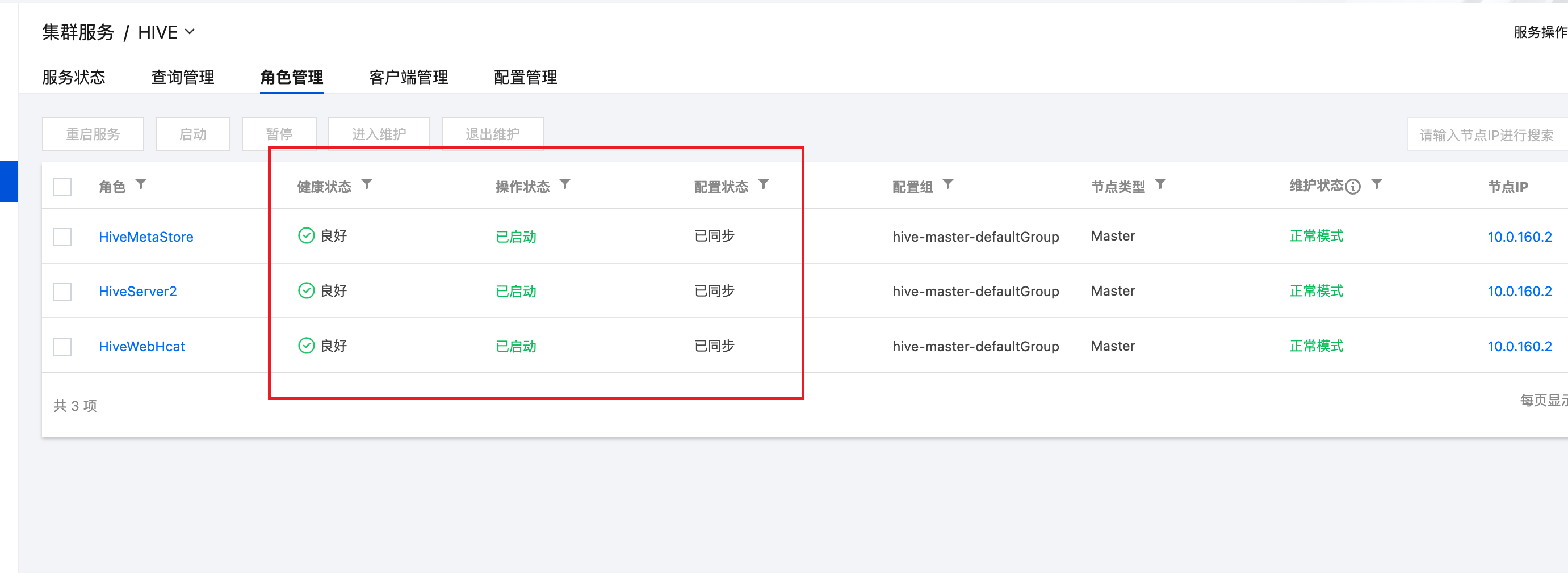
- (If Kerberos authentication is not enabled, skip this step) Add Kerberos users and export the authentication-related configurations. Log in to the EMR Master machine and run the following commands as root to generate the authentication configuration files.
$ kadmin.local
addprinc -pw ${input your password} ${input your Principal}
ktadd -k /root/test.keytab ${input your Principal}
exit
cp /etc/krb5.conf ~/krb5.conf
# Modify ~/krb5.conf to Add `allow_weak_crypto = True` Under Libdefaults (because the Tencent Cloud Deployment of Kerberos Uses the Insecure Des3-cbc-sha1 Algorithm).
vim ~/krab5.conf
# In the Integration Setup Below, the Krb5.conf and Test.keytab Files Will Be Used.
-
(If Kerberos authentication is not enabled, you may skip this step) Go to the configuration management page for the Hive service and retrieve the following configuration values from the
hive-site.xml
file:-
hive.metastore.uris
, as the User Principal for the subsequent steps. -
hive.metastore.kerberos.principal
, as the Kerberos Principal for the subsequent steps.
-
Step 2: Create Hive Catalog Integration
After the Hive Catalog service is created, you need to go to the AutoMQ console to create a Hive Catalog integration and enter the Catalog information configured in step 1. The operations are as follows:
- Log in to the AutoMQ console and click on the Integration menu.
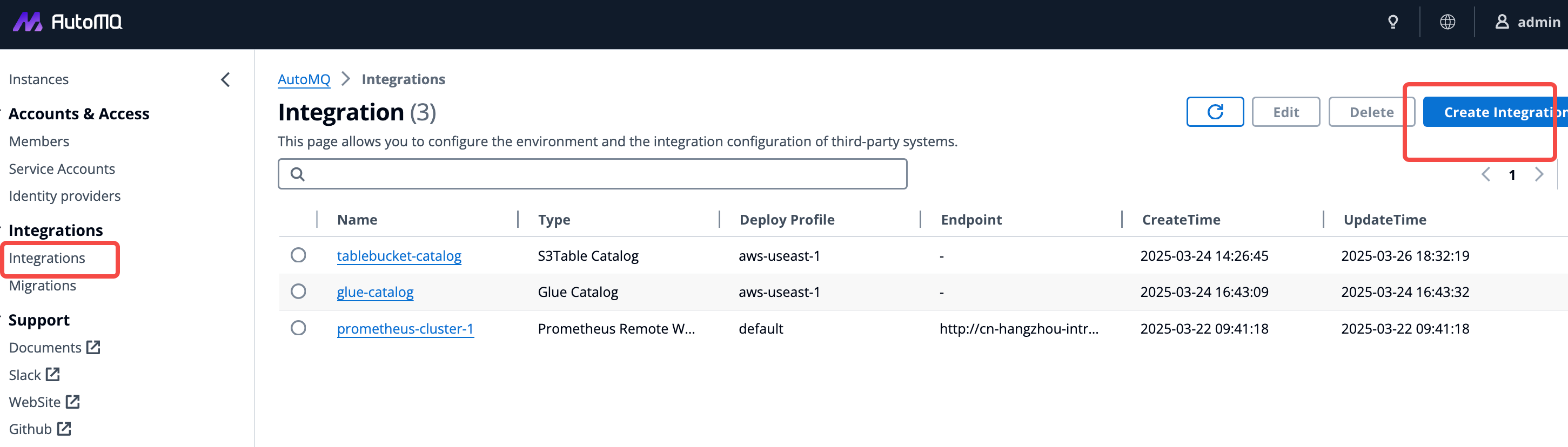
-
Select Create Hive Catalog Integration and fill in the following information:
-
Name: Enter a distinctive integration configuration name.
-
Deployment Configuration: Select the deployment configuration to which the integration belongs; subsequent instance creation must be consistent.
-
Hive Metastore Access Point: Set up the Hive Metastore access point; AutoMQ will query the Data Catalog through this access point.
-
Authentication Type: Select either No Authentication or Kerberos Authentication based on your requirements.
-
KeyTab File: If you have enabled Kerberos Authentication, upload the KeyTab file generated in step 1.
-
Krb5.conf File: If you have enabled Kerberos Authentication, upload the Krb5.conf file generated in step 1.
-
Kerberos User Principal: If you have enabled Kerberos Authentication, input the User Principal obtained in step 1.
-
Kerberos Principal: If Kerberos authentication is enabled, input the Kerberos Principal obtained in Step 1.
-
Warehouse: Enter the object storage Bucket used by your data lake. This Bucket is designated for long-term data storage.
-
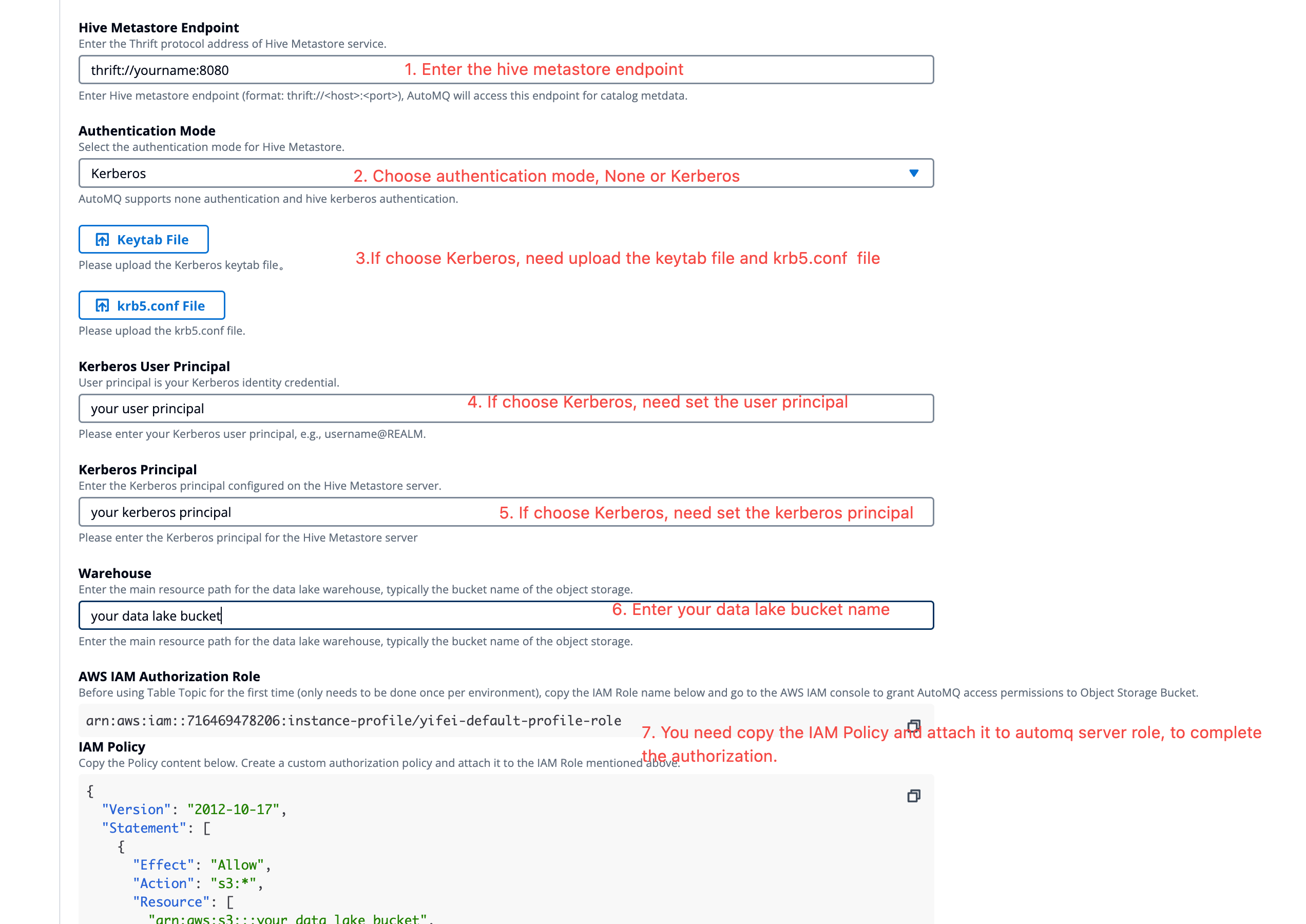
-
After inputting the Warehouse parameters, AutoMQ will generate the CAM Policy required to access this Bucket and display the IAM Role currently utilized by the AutoMQ instance. Please refer to the Policy to create the authorization in the IAM console of your Cloud providers.
-
Once the authorization is created, you can proceed to create the Hive Catalog integration.
Step 3: Create an AutoMQ Instance and Enable the Table Topic Feature
To use the AutoMQ Table Topic feature, you need to enable it during instance creation to stream data into the lake subsequently. Please refer to the instructions below for configuring instance creation:
Note:
Even after enabling Table Topic for the AutoMQ instance, not all Topics will default to stream tables. Each Topic must be configured individually to achieve streaming data into the lake.
To use Table Topic, it must be enabled during instance creation. Once the instance is created, this configuration cannot be changed.
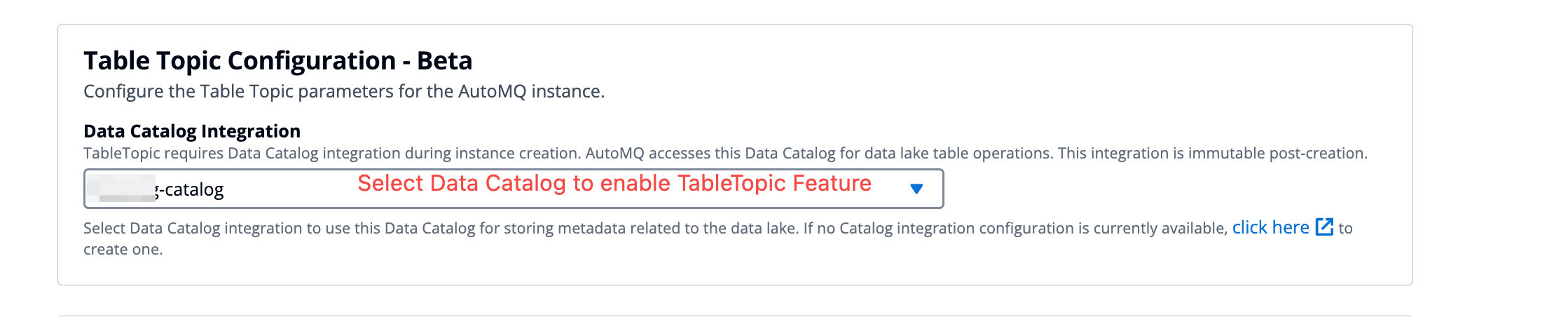
Step 4: Create a Topic and Configure Stream Tables
After enabling the Table Topic feature for an AutoMQ instance, you can configure the streaming table as needed when creating a Topic. The specific steps are as follows:
-
Navigate to the instance from step 3, go to the Topic list, and click on Create Topic.
-
In the configuration settings for Topic creation, enable the Table Topic conversion and configure the following parameters:
-
Namespace: The namespace is used to isolate different Iceberg tables and corresponds to the Database in the Data Catalog. It is recommended to set the parameter value based on business attributes.
-
Schema constraint type: Set whether Topic messages follow Schema constraints. If you select 'Schema', Schema constraints are enforced, requiring the registration of message Schemas in the AutoMQ built-in SchemaRegistry, where messages sent later must strictly adhere to the Schema, and subsequent Table Topic will use the Schema fields to populate the Iceberg table; if you select 'Schemaless', it indicates that the message content is not bound by any strict Schema constraints, and the message Key and Value will be populated as whole fields into the Iceberg table.
-
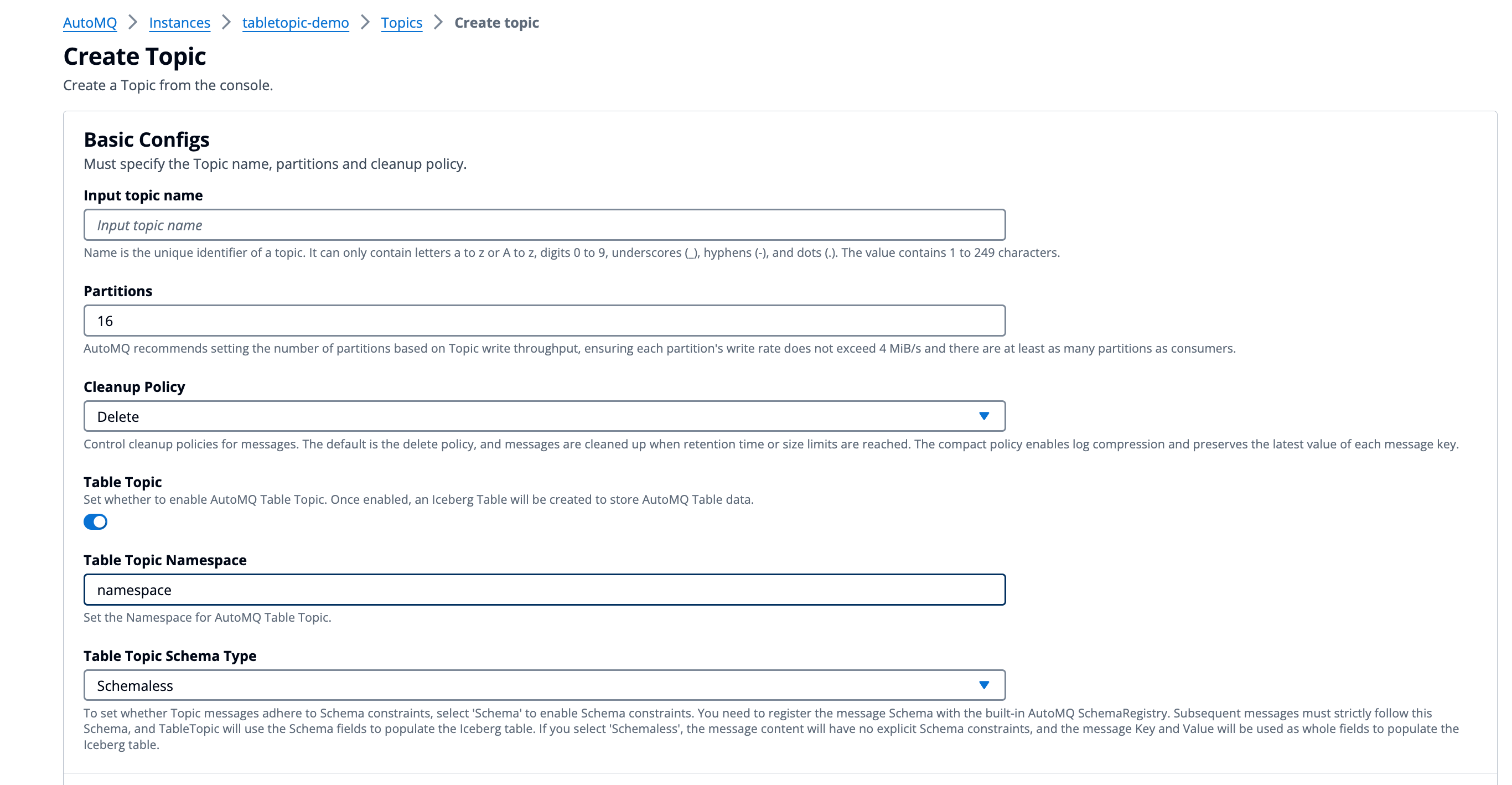
- Click Confirm to create a Topic that supports flow tables.
Step 5: Produce Messages and Query Iceberg Table Data Using Spark SQL
After completing the AutoMQ instance configuration and creating the Table Topic, you can proceed to test data production and query data in the Iceberg table.
- Click to enter Topic details, go to the message production tab, input the test message Key and message Value, and send the message.
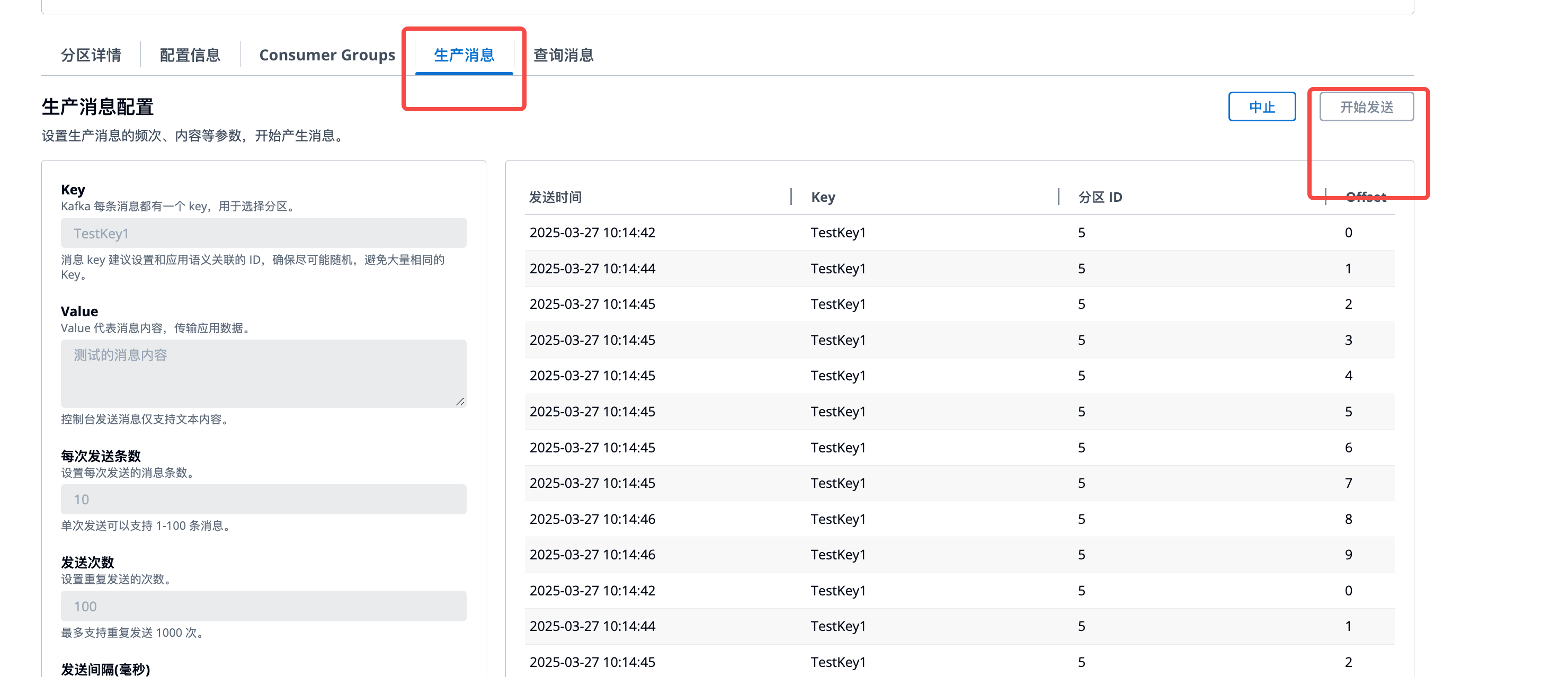
-
Add the Spark component, then log in to the EMR cluster's Master node and switch to the hadoop user.
-
Execute the following command to enter spark-sql.
export HIVE_URI="thrift://YOUR_HOST:7004"
export WAREHOUSE="s3a://YOUR_BUCKER/iceberg"
export AWS_ACCESS_KEY=
export AWS_SECRET_KEY=
export AWS_REGION=
export AWS_S3_ENDPOINT='https://cos.$AWS_REGION.myqcloud.com'
spark-sql --master local[*] \
--conf spark.sql.extensions=org.apache.iceberg.spark.extensions.IcebergSparkSessionExtensions \
--conf spark.sql.catalog.hive=org.apache.iceberg.spark.SparkCatalog \
--conf spark.jars.packages=org.apache.iceberg:iceberg-spark-runtime-3.2_2.12:0.13.1,software.amazon.awssdk:bundle:2.31.14,org.apache.hadoop:hadoop-aws:3.3.1,software.amazon.awssdk:url-connection-client:2.17.178 \
--conf spark.sql.defaultCatalog=hive \
--conf spark.sql.catalog.hive.type=hive \
--conf spark.sql.catalog.hive.uri=$HIVE_URI \
--conf spark.sql.catalog.hive.io-impl=org.apache.iceberg.aws.s3.S3FileIO \
--conf spark.sql.catalog.hive.s3.endpoint=$AWS_S3_ENDPOINT \
--conf spark.sql.catalog.hive.warehouse=$WAREHOUSE \
--conf spark.sql.catalog.hive.client.region=$AWS_REGION \
--conf spark.sql.catalog.hive.s3.path-style-access=false \
--conf spark.sql.catalog.hive.s3.access-key-id=$AWS_ACCESS_KEY \
--conf spark.sql.catalog.hive.s3.secret-access-key=$AWS_SECRET_KEY \
--conf spark.hadoop.fs.s3a.path.style.access=false \
--conf spark.hadoop.fs.s3a.region=$AWS_REGION
- Execute SQL queries. You will see that AutoMQ converts Kafka Messages into corresponding data records in real time. Users can also use other query engines for analysis and computation.
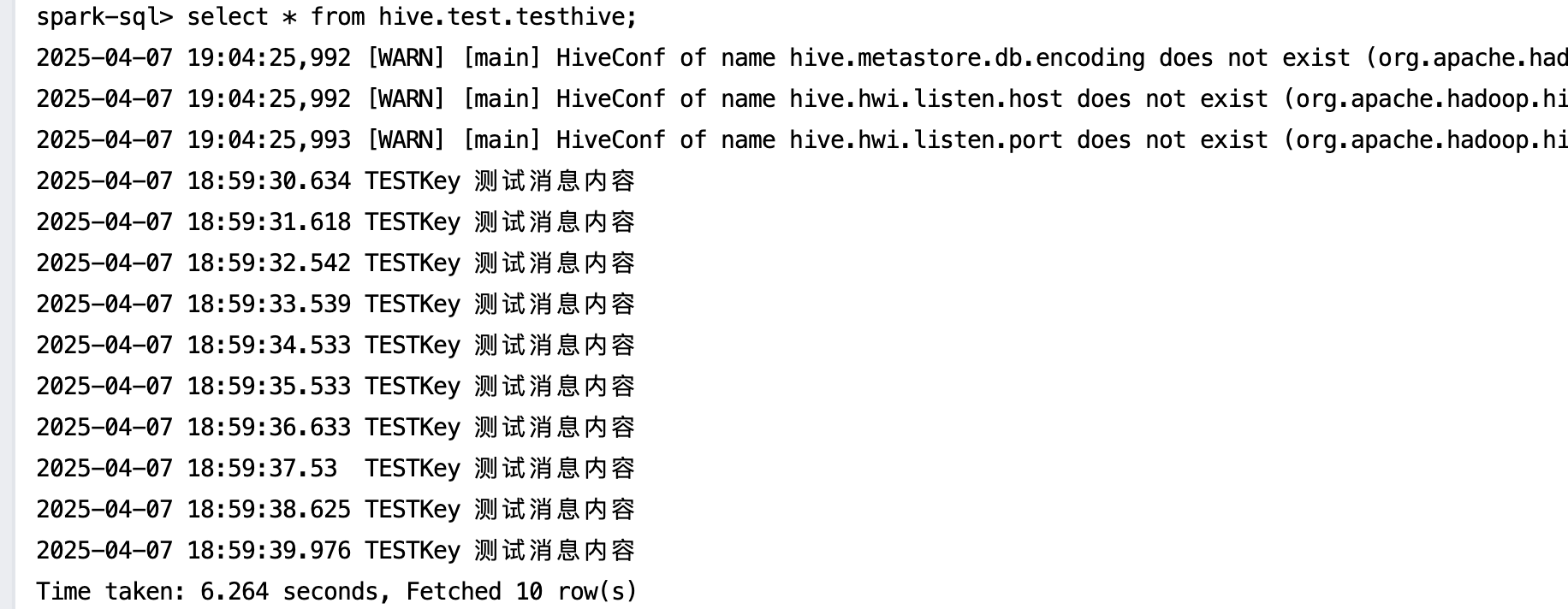