Overview
Amazon Web Services (AWS) offers multiple messaging and streaming services, with Amazon Kinesis and Amazon Simple Queue Service (SQS) being two of the most widely used options. While both facilitate data movement between application components, they serve fundamentally different purposes and excel in different scenarios. This comprehensive comparison explores their key differences, use cases, and technical considerations.
Before diving into the detailed comparison, the key finding is that Kinesis is optimized for real-time, high-volume data streaming with multiple consumers, while SQS excels at reliable message queuing for decoupling application components with simpler operational requirements.
Core Concepts and Architecture
Amazon Kinesis
Kinesis is a platform for streaming data on AWS, allowing real-time processing of high-volume data streams[1]. It's built on the concept of persistent data streams composed of shards, which represent the base throughput unit.
Key components include:
Data Streams : Core service for capturing and storing streaming data
Shards : Base throughput units (1MB/sec input, 2MB/sec output per shard)
Records : Data units with partition keys for distribution across shards
Retention : Data persists for 24 hours by default, configurable up to 365 days[15]
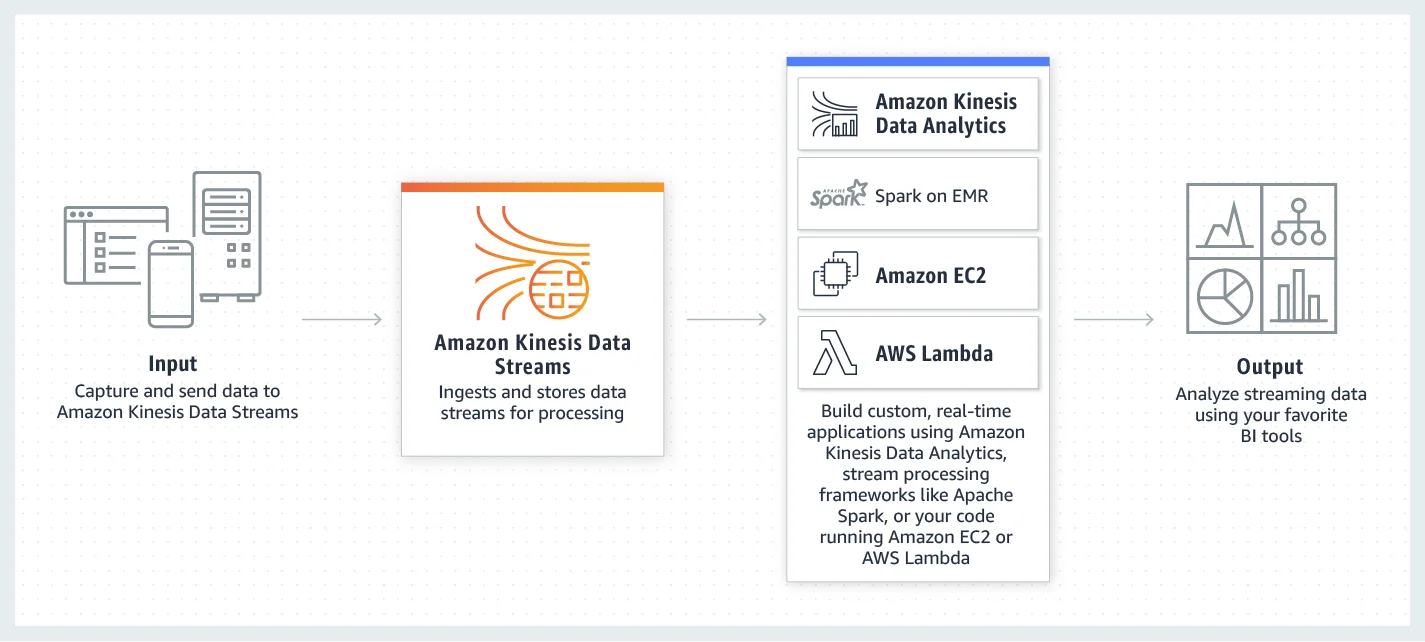
Amazon SQS
SQS is a fully managed message queuing service designed to decouple and scale microservices, distributed systems, and serverless applications[1]. It provides a simple queue model with guaranteed at-least-once delivery.
Key components include:
Queues : Standard (high throughput) or FIFO (ordered delivery)
Messages : Individual data units (up to 256KB each)
Visibility Timeout : Period during which messages are invisible after being retrieved
Retention : Messages persist up to 4 days by default, configurable from 1 minute to 14 days[15]
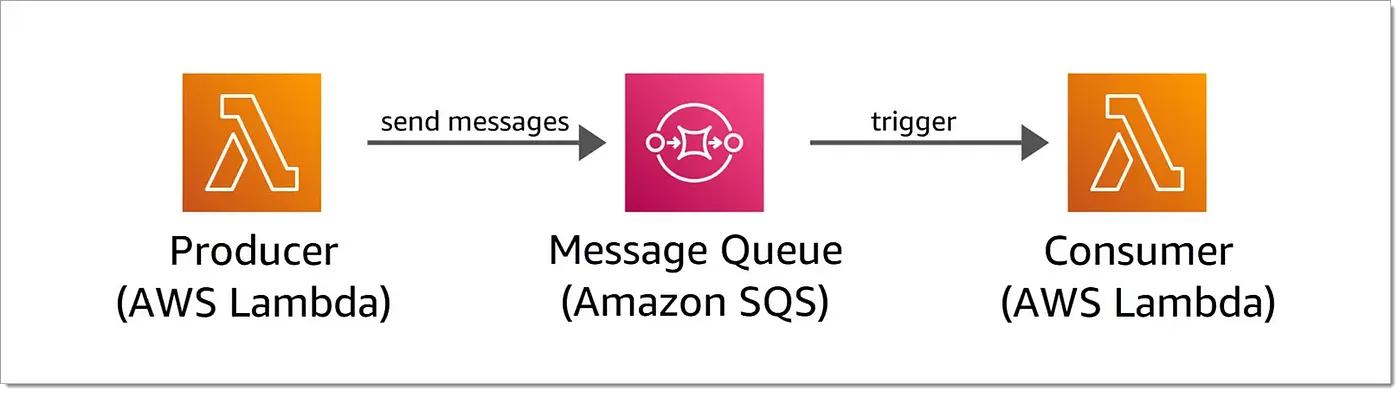
Key Differences
Purpose and Design Philosophy
Aspect | Kinesis | SQS |
---|---|---|
Primary Purpose | Real-time data streaming and analytics | Message queuing and application decoupling |
Design Focus | High-volume streaming data processing | Reliable message delivery between components |
Processing Model | Stream processing with multiple consumers | Queue processing with individual consumers |
Data Lifecycle | Persistent with configurable retention | Deleted after successful processing |
Kinesis is primarily designed for real-time data streaming applications that need to process and analyze large volumes of continuous data[1]. SQS, on the other hand, focuses on reliable message queuing to decouple application components and ensure message delivery[3].
Data Model and Consumption Patterns
Kinesis maintains data streams that can be read by multiple consumers simultaneously, allowing for different applications to process the same data independently[10]. With SQS, each message is typically processed by a single consumer and then deleted from the queue[2].
Message Ordering and Delivery Guarantees
Kinesis preserves the ordering of records at the shard level, meaning records with the same partition key will be processed in order[10]. SQS offers two queue types:
Standard queues : High throughput with at-least-once delivery but no guaranteed order
FIFO queues : Exactly-once processing with guaranteed ordering but lower throughput[15]
Scalability Characteristics
Feature | Kinesis | SQS |
---|---|---|
Scaling Model | Manual provisioning or on-demand | Fully automatic |
Throughput Limits | 1MB/s in, 2MB/s out per shard | 3,000 msgs/sec standard, 30,000 msgs/sec high throughput |
Maximum Message Size | 1MB | 256KB |
Operational Overhead | Higher (shard management) | Lower (fully managed) |
Kinesis requires explicit capacity planning through shard provisioning, whereas SQS scales automatically to match demand[1][5]. However, Kinesis can achieve higher overall throughput with appropriate shard allocation[5].
Use Cases
When to Use Kinesis
Real-time Analytics : Processing streaming data for immediate insights[7]
IoT Data Processing : Handling large-scale data from IoT devices[1]
Log and Event Data Collection : Centralized collection of logs and events[5]
Clickstream Analysis : Processing website user activity in real-time[7]
Multiple Consumer Applications : When multiple applications need to process the same data[1]
When to Use SQS
Application Decoupling : Separating components of distributed applications[7]
Task Queues : Managing job processing and workload distribution[10]
Batch Processing : Queuing items for batch processing workflows[3]
Microservice Communication : Reliable messaging between microservices[3]
Load Leveling : Smoothing out traffic spikes to backend systems[1]
Hybrid Approaches
Some architectures benefit from using both services together:
Using Kinesis for initial high-volume data capture and SQS for specific processing tasks[6]
Implementing Kinesis for real-time processing and SQS for task distribution to workers[6]
Implementation Details
Configuration and Management
Kinesis Configuration
## AWS CLI example for creating a Kinesis stream
aws kinesis create-stream --stream-name MyDataStream --shard-count 5
Considerations:
Shard count determines throughput capacity and cost
Partition key design affects data distribution across shards
Enhanced fan-out for high-demand consumers requires explicit configuration
Consumer applications often use Kinesis Client Library (KCL) with DynamoDB for checkpointing[10]
SQS Configuration
## AWS CLI example for creating an SQS queue
aws sqs create-queue --queue-name MyQueue --attributes DelaySeconds=0,MaximumMessageSize=262144
Considerations:
Queue type selection (Standard vs. FIFO) based on ordering requirements
Appropriate visibility timeout to prevent duplicate processing
Dead-letter queue configuration for handling failed message processing
Message retention period based on application requirements
Integration with AWS Ecosystem
Kinesis Integrations
Kinesis integrates seamlessly with many AWS services:
Lambda : For serverless stream processing
Firehose : For delivery to S3, Redshift, Elasticsearch, or Splunk
Analytics : For SQL queries against streaming data
Data Warehouse Services : Direct integration with Redshift and S3[1]
SQS Integrations
SQS works well with:
Lambda : Direct invocation when messages arrive
EC2/ECS : For traditional worker patterns
Step Functions : For complex workflows
EventBridge : For event-driven architectures[5]
Best Practices
Kinesis Best Practices
Use shard-level metrics to monitor performance and identify hotspots[14]
Monitor IteratorAge metric to prevent data loss from expired iterators[14]
Implement proper exception handling for "poison messages" that can cause batch failures[14]
Design partition keys to distribute data evenly across shards
Consider enhanced fan-out for high-throughput consumers[6]
SQS Best Practices
Configure appropriate visibility timeout based on expected processing time
Implement dead-letter queues to capture and analyze failed messages
Use batch operations (SendMessageBatch, ReceiveMessage with MaxNumberOfMessages) for efficiency
Implement exponential backoff for handling throttling conditions
Consider long polling to reduce empty responses and API calls[5]
Cost and Comparison
Pricing and Cost Optimization
Aspect | Kinesis | SQS |
---|---|---|
Pricing Model | Pay per shard-hour or on-demand | Pay per million requests |
Small Volume Cost | Higher cost floor | More cost-effective |
Large Volume Cost | More efficient at very high throughput | Can become expensive at extreme scales |
Cost Estimation | Based on shard count and data volume | Based on request count and retention |
At small data volumes (1GB/day), SQS is significantly less expensive ($0.20/month vs. $10.82/month for Kinesis)[2]. However, as volume increases to 1TB/day, Kinesis becomes more cost-effective ($158/month vs. $201/month for SQS)[2].
Comparison with Alternative Solutions
Kinesis vs. Confluent (Kafka)
Aspect | Kinesis | Confluent |
---|---|---|
Focus | Streaming data service | Complete streaming platform |
Data Model | Streams & Shards | Distributed commit logs (topics) |
Retention | Up to 365 days | Unlimited potential retention |
Ecosystem | AWS services integration | Rich connector ecosystem |
Management | Fully managed by AWS | Self-managed or Confluent Cloud |
Confluent offers greater flexibility and unlimited retention compared to Kinesis' maximum 365-day retention[3][9]. However, Kinesis provides tighter integration with AWS services and lower operational overhead[3].
Kinesis vs. Redpanda
Redpanda offers higher performance than Kinesis with fewer resources due to its C++ foundation and efficient design[4][12]. It excels in self-hosted environments with three times fewer nodes than traditional Kafka setups[12]. However, Kinesis benefits from being a fully managed AWS service with automatic scaling and tight AWS ecosystem integration[4].
Decision Framework
When choosing between these services, consider:
Data Characteristics :
Volume: High-volume streaming data favors Kinesis
Ordering: If strict ordering is required, use Kinesis or SQS FIFO
Retention: Long retention needs favor Kinesis
Consumption Pattern :
Single consumer: SQS is simpler
Multiple consumers: Kinesis allows multiple applications to process the same data
Operational Preferences :
Fully managed with minimal configuration: SQS
Control over scaling and processing: Kinesis
Integration Requirements :
Deep AWS integration: Both work well
Event-driven architectures: SQS often simpler
Real-time analytics pipeline: Kinesis preferred
Conclusion
Amazon Kinesis and SQS serve different but complementary purposes in distributed architectures. Kinesis excels at high-volume, real-time data streaming with multiple consumers, while SQS provides simple, reliable message queuing for decoupling application components.
Many modern architectures leverage both: Kinesis for capturing and processing high-volume streaming data and SQS for reliable task distribution and application decoupling. Understanding their distinct characteristics and selecting the right service (or combination) for your specific use case is essential for building efficient, scalable, and cost-effective cloud-native applications.
If you find this content helpful, you might also be interested in our product AutoMQ. AutoMQ is a cloud-native alternative to Kafka by decoupling durability to S3 and EBS. 10x Cost-Effective. No Cross-AZ Traffic Cost. Autoscale in seconds. Single-digit ms latency. AutoMQ now is source code available on github. Big Companies Worldwide are Using AutoMQ. Check the following case studies to learn more:
Grab: Driving Efficiency with AutoMQ in DataStreaming Platform
Palmpay Uses AutoMQ to Replace Kafka, Optimizing Costs by 50%+
How Asia’s Quora Zhihu uses AutoMQ to reduce Kafka cost and maintenance complexity
XPENG Motors Reduces Costs by 50%+ by Replacing Kafka with AutoMQ
Asia's GOAT, Poizon uses AutoMQ Kafka to build observability platform for massive data(30 GB/s)
AutoMQ Helps CaoCao Mobility Address Kafka Scalability During Holidays
JD.comx AutoMQ x CubeFS: A Cost-Effective Journey at Trillion-Scale Kafka Messaging
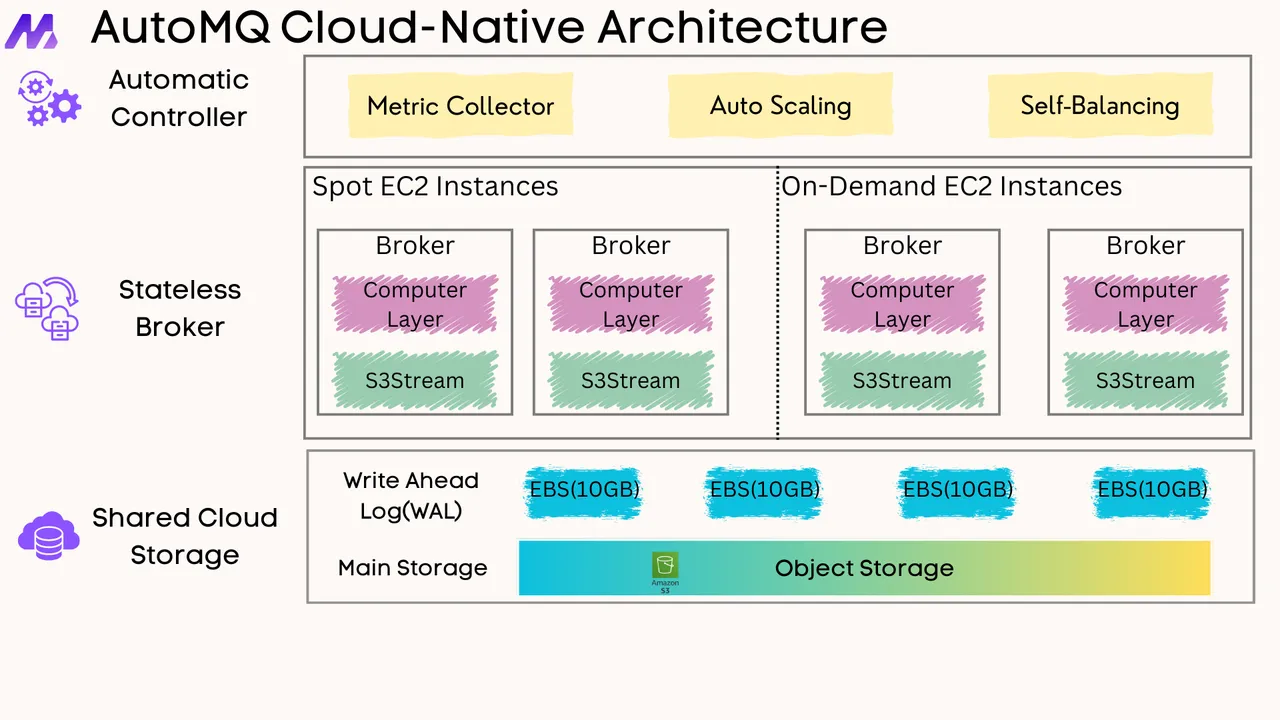
References:
Why should I use Amazon Kinesis and not SNS/SQS? - Stack Overflow
Architectural Guidance for IoT Workload with SQS and Kinesis - AWS re:Post
Differences Between Kinesis Data Stream and SQS - Classmethod
Event-Driven Architecture Best Practices - AWS Architecture Blog
Mastering Retries: Amazon SQS Best Practices - RanTheBuilder