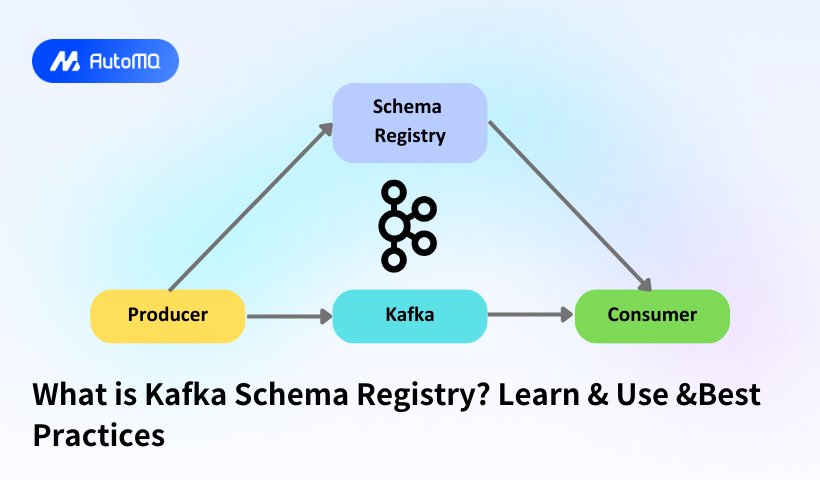
Overview
Kafka Schema Registry serves as a centralized repository for managing and validating schemas used in data processing and serialization. This comprehensive guide explores how Schema Registry works, its architecture, configuration options, and best practices to ensure data consistency and compatibility in Kafka ecosystems.
What is Kafka Schema Registry?
Schema Registry provides a RESTful interface for storing and retrieving schemas (Avro, JSON Schema, and Protobuf) used by Kafka producers and consumers. In Kafka, messages are simply transferred as byte arrays, with no inherent understanding of the data structure. Schema Registry addresses this limitation by maintaining a versioned history of schemas and enabling schema evolution according to configured compatibility settings[1][13].
The Schema Registry acts as the central source of truth for all schema information and schema-to-ID mappings, ensuring that:
Producers validate data against registered schemas before sending it to Kafka topics
Consumers can accurately deserialize incoming messages using the correct schema version
Data format changes can be managed in a controlled, compatible manner
Applications can evolve independently without breaking downstream consumers[13][19]
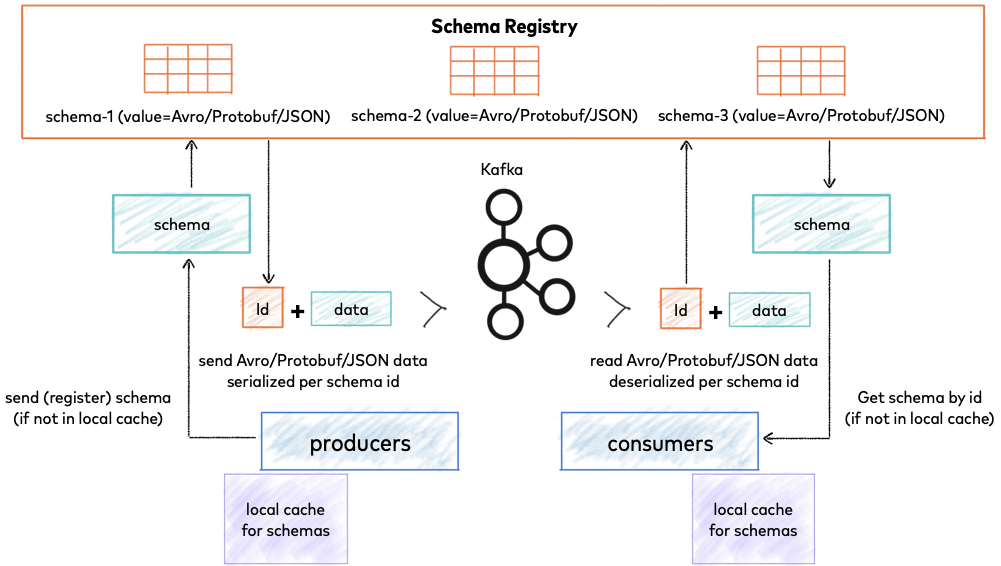
How Schema Registry Works
When integrated with Kafka clients, Schema Registry follows a specific workflow[1][13][18]:
Producer Registration : Before sending data, a producer checks if its schema is already registered in Schema Registry. If not, it registers the schema and receives a unique schema ID.
Message Serialization : The producer serializes the data according to the schema and embeds the schema ID (not the entire schema) in the message payload.
Message Transmission : The serialized data with the schema ID is sent to Kafka.
Consumer Deserialization : When a consumer receives a message, it extracts the schema ID from the payload, fetches the corresponding schema from Schema Registry, and uses it to deserialize the data.
Schema Caching : Both producers and consumers cache schemas locally to minimize Schema Registry calls, only contacting it when encountering new schema IDs[1].
The schema ID in the message payload follows a specific wire format, which includes a magic byte, schema ID, and the actual serialized data[1].
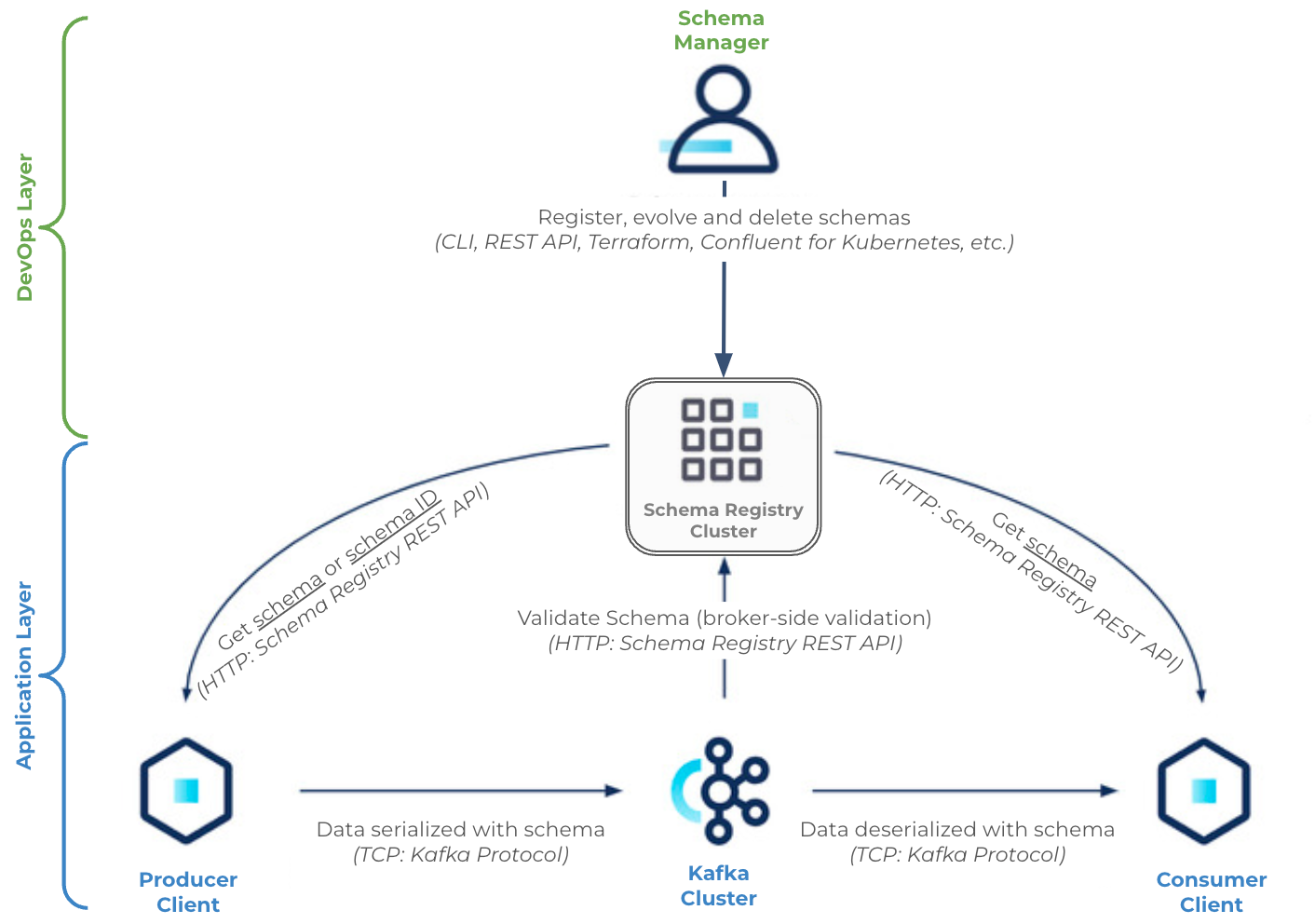
Schema Registry Architecture
Schema Registry is designed as a distributed service with a single primary architecture[14]:
Single Primary Architecture
Only one Schema Registry instance serves as the primary at any time
Only the primary can write to the underlying Kafka log (the schemas topic)
All nodes can directly serve read requests
Secondary nodes forward write requests to the primary[14]
Primary election can occur through two mechanisms:
Kafka Group Protocol (recommended): Uses Kafka's coordination for leader election
ZooKeeper (deprecated): Uses ZooKeeper for leader election[14]
Different vendors implement Schema Registry with variations:
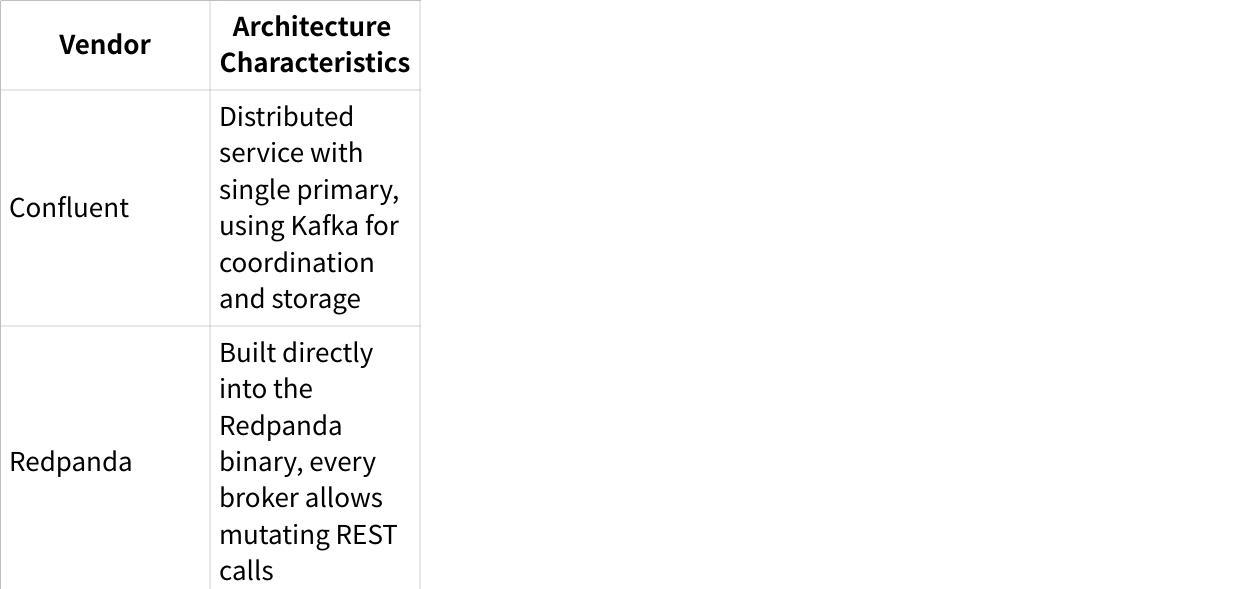
Key Components and Concepts
Subjects and Naming Strategies
Subjects provide a unique namespace for organizing schemas. The subject naming strategy determines how schemas are associated with topics[11][13]:
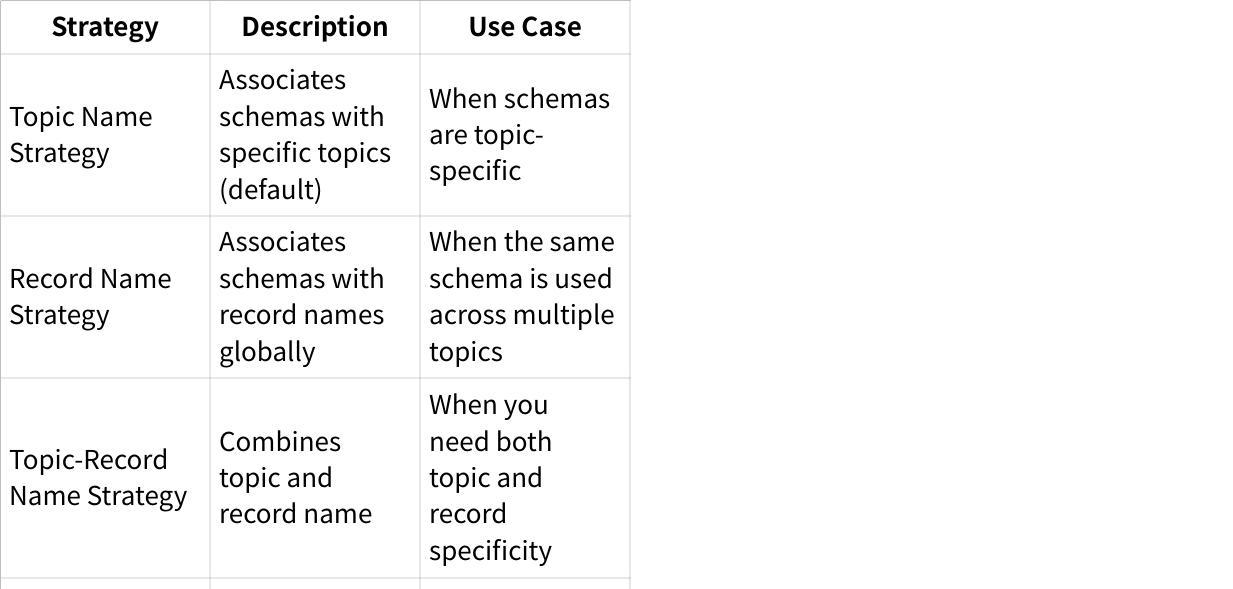
Compatibility Modes
Schema Registry supports different compatibility modes to control schema evolution[13]:
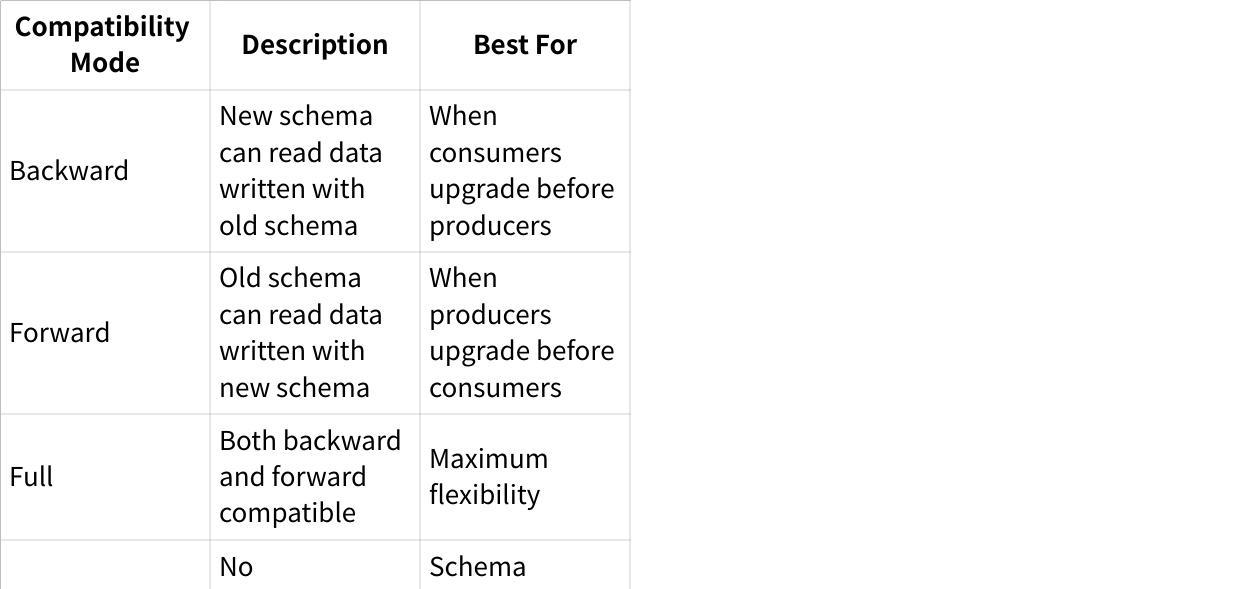
Configuration Best Practices
Security Configuration
Enable encryption : Configure SSL/TLS for Schema Registry connections
Implement authentication : Set up SASL mechanisms for client authentication
Configure authorization : Restrict who can register or modify schemas
Use HTTPS : Enable HTTPS for REST API calls instead of HTTP[10]
High Availability Setup
Deploy multiple instances : For redundancy and high availability
Use a virtual IP (VIP) : Place in front of Schema Registry instances for easier client management
Consistent configuration : Ensure all instances use the same schemas topic name
Unique host names : Configure different host.name values for each instance[10]
Schemas Topic Configuration
Use compaction : Ensure the schemas topic has a compact retention policy
Adequate replication : Configure with a replication factor of at least 3
Protection : Protect the schemas topic from accidental deletion[10][12]
Common Pitfalls and How to Avoid Them
Based on industry experience, here are the most common Schema Registry issues and their solutions[10]:
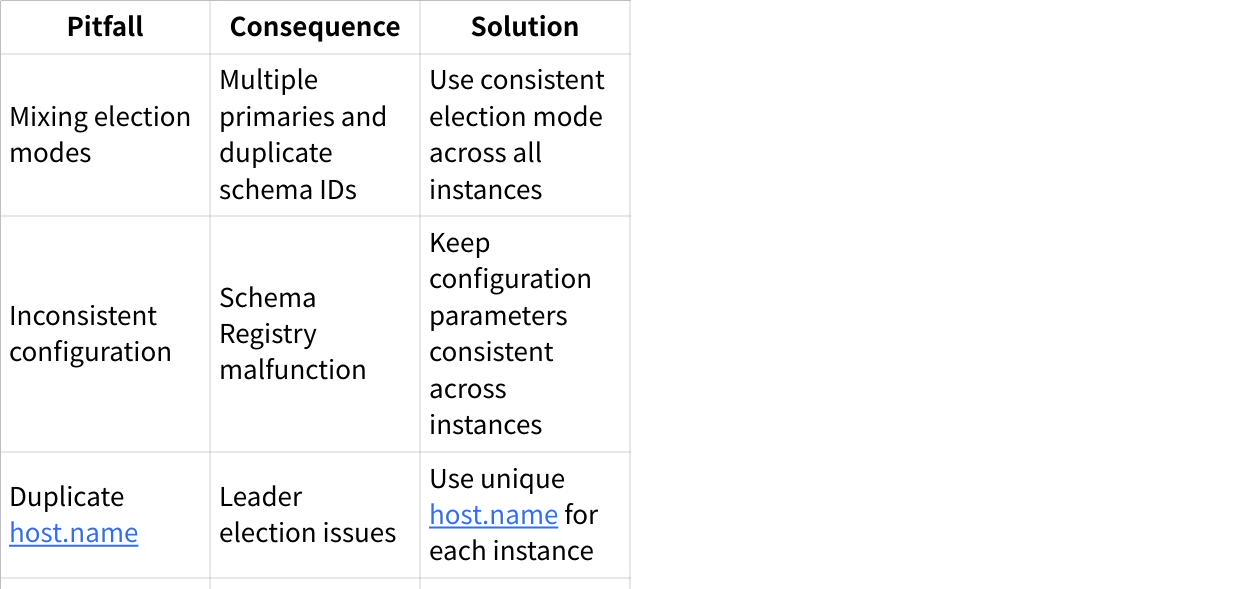
Schema Evolution Best Practices
When evolving schemas, follow these guidelines to ensure compatibility[13]:
- Provide default values for all fields that might be removed in the future
- Never rename existing fields instead add aliases to maintain compatibility
Never delete required fields from schemas
Add fields with default values to maintain backward compatibility
Create new topics (with -v2 suffix) for complete schema rewrites[1][13]
Client Integration
Clients integrate with Schema Registry through serializers and deserializers (SerDes)[1][7]:
// Producer configuration example
props.put("key.serializer", "io.confluent.kafka.serializers.KafkaAvroSerializer");
props.put("value.serializer", "io.confluent.kafka.serializers.KafkaAvroSerializer");
props.put("schema.registry.url", "http://schema-registry:8081");
// Consumer configuration example
props.put("key.deserializer", "io.confluent.kafka.serializers.KafkaAvroDeserializer");
props.put("value.deserializer", "io.confluent.kafka.serializers.KafkaAvroDeserializer");
props.put("schema.registry.url", "http://schema-registry:8081");
props.put("specific.avro.reader", "true");
Management Tools
Several tools help manage Schema Registry effectively[2][11][16]:
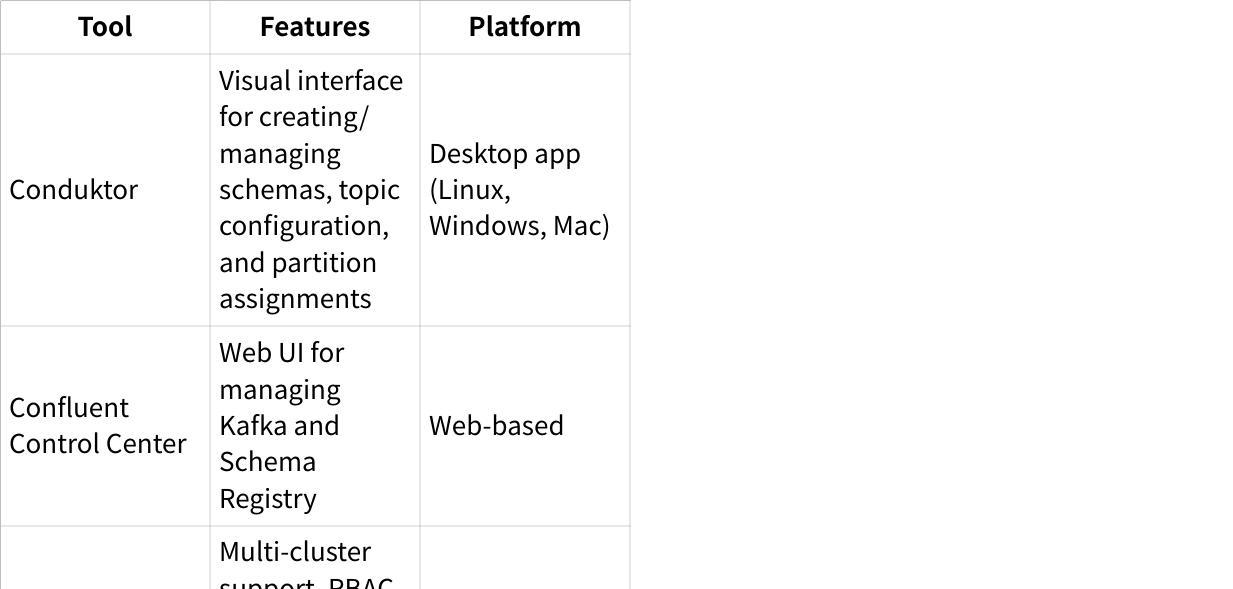
When to Use Schema Registry
Schema Registry is most valuable when[13]:
Schema changes are expected in the future
Data needs to adhere to standardized formats
Multiple teams or applications interact with the same data
You need to enforce data quality and validation
However, it might not be necessary when schemas are fixed and won't change, or when using simple data formats with minimal structure.
Conclusion
Kafka Schema Registry provides essential functionality for maintaining data consistency and compatibility in event-driven architectures. By centralizing schema management, enforcing compatibility rules, and enabling controlled schema evolution, it helps organizations build robust, maintainable data pipelines. Following the configuration and evolution best practices outlined in this guide will help you avoid common pitfalls and leverage Schema Registry effectively in your Kafka ecosystem.
If you find this content helpful, you might also be interested in our product AutoMQ. AutoMQ is a cloud-native alternative to Kafka by decoupling durability to S3 and EBS. 10x Cost-Effective. No Cross-AZ Traffic Cost. Autoscale in seconds. Single-digit ms latency. AutoMQ now is source code available on github. Big Companies Worldwide are Using AutoMQ. Check the following case studies to learn more:
Grab: Driving Efficiency with AutoMQ in DataStreaming Platform
Palmpay Uses AutoMQ to Replace Kafka, Optimizing Costs by 50%+
How Asia’s Quora Zhihu uses AutoMQ to reduce Kafka cost and maintenance complexity
XPENG Motors Reduces Costs by 50%+ by Replacing Kafka with AutoMQ
Asia's GOAT, Poizon uses AutoMQ Kafka to build observability platform for massive data(30 GB/s)
AutoMQ Helps CaoCao Mobility Address Kafka Scalability During Holidays
JD.com x AutoMQ x CubeFS: A Cost-Effective Journey at Trillion-Scale Kafka Messaging
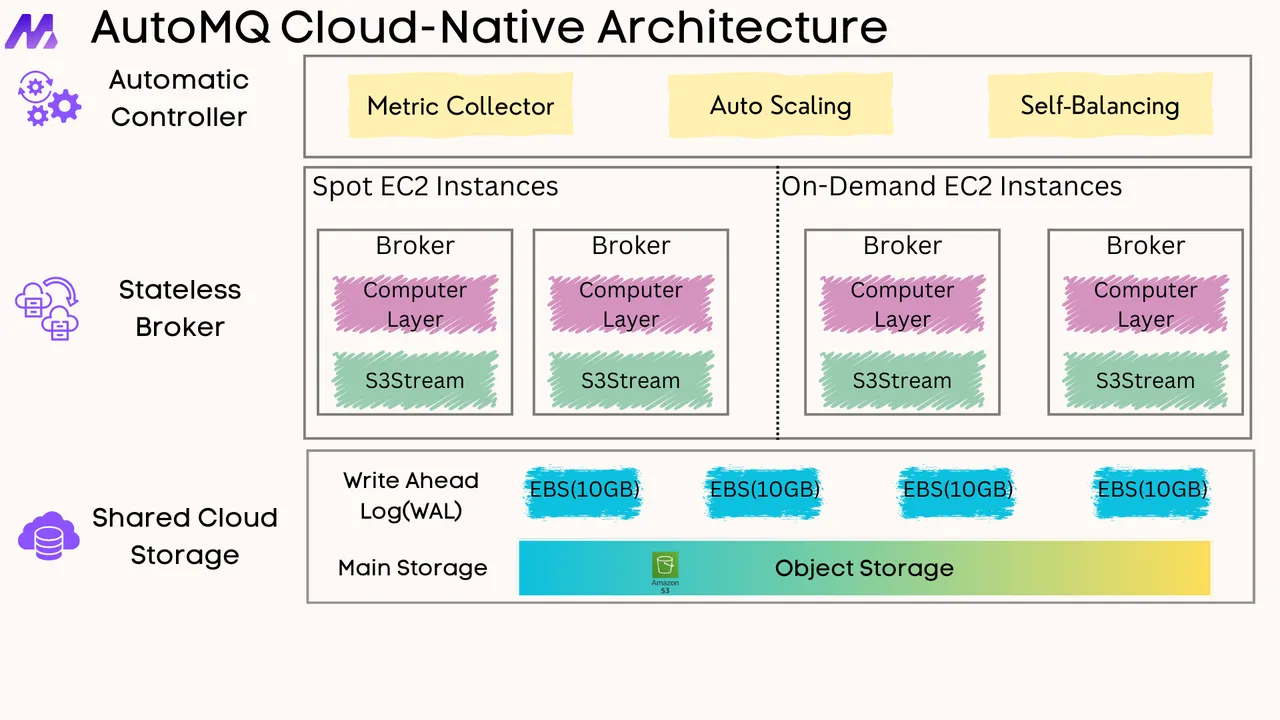
References:
Implementing Schema Registry in Confluent Kafka: Best Practices and Use Cases
How to Connect Redpanda Cluster and Topic to Redpanda Schema Registry?
Looking for Resources on Kafka/Confluent CI/CD Best Practices
Data Quality and Policy Enforcement for Apache Kafka with Schema Registry
Schema Registry IDs are Different from What's in the Registry